A guide for selecting the level of significance
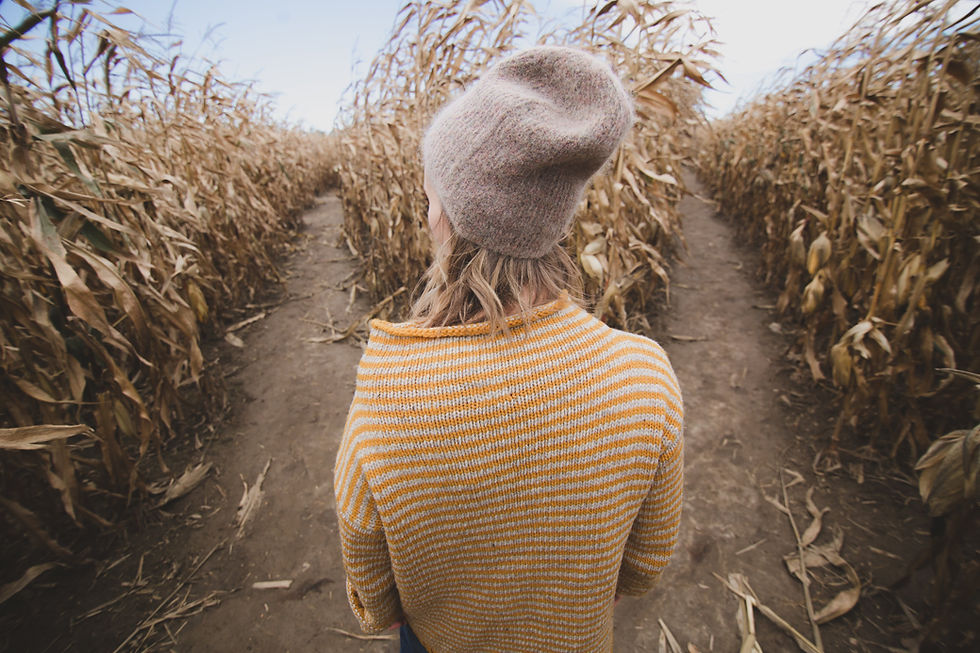
In hypothesis testing, the “p-value < α” is almost universally used as a criterion for statistical significance and also as a decision rule, where α is the level of significance. For example, in testing for
H0: θ = 0; H1: θ ≠ 0,
where θ is the parameter of interest that represents an effect, we reject H0 of no effect, at the α level of significance, if p-value < α. It is a convention to set α = 0.05, while 0.10 and 0.01 are also widely adopted. As it is well-known, these conventional values are arbitrary and have little scientific justification.
When the p-value is close to 0.05, what do you have to do? For example,
if p-value = 0.052, should you maintain α = 0.05 and accept H0? Or, should you set α = 0.10 and reject it?
Similarly, if the p-value = 0.047, should you maintain α = 0.05 and reject H0? Or, should you set α = 0.01 and accept H0?
Statistical textbooks provide little guidance on this point, while such situations are frequently encountered in practical applications of statistical analysis and machine learning methods. The aim of this post is to provide the researchers with a few key points to consider in reaching a rational decision, when they are faced with this situation. While the discussion below is applicable to any p-value close to 0.05, let us assume that p-value = 0.052 for the purpose of illustration, without loss of generality. Two real-world examples are provided as applications.
1. Meaning of setting the value of α
The level of significance α represents the probability of Type I error (rejection of a true H0). Setting a low value of α means that you want to control the Type I error with a low probability.
In principle, the choice for the level of significance should be made in consideration of the probability of Type II error (acceptance of a false H0), denoted β; statistical power (1-β); sample size; and losses from Type I and II errors, before the data is collected. Please see this post for details.
A trade-off between α and β is well-known. A higher value of α leads to a lower value β; and vice versa. Setting a high value of α means that you want to control the Type II error with a low probability.
Both error probabilities cannot be lowered at the same time, as long as the sample size is fixed.
2. What not to do
Turning back to the situation where the p-value is close to 0.05, we begin by answering the question of what not to do.
The most unscientific way is to adjust the value of α to meet or justify your preconceived decision. That is, the value of α should not be chosen “on the desire of an investigator to reject or accept a hypothesis”: see Keuzenkamp and Magnus (1995, p. 20). As an example, consider the case where p-value = 0.052. Suppose the researcher has initiated the research with an aim or intention to reject H0, so she chooses α = 0.10 and rejects H0. Conversely, if the researcher was intended to accept H0, then she chooses α = 0.01 and accepts H0. The above is unscientific and arbitrary, and even be unethical. This process is called p-hacking or data snooping, which is a major cause of the accumulation of incorrect stylized facts in many fields of science: see the statement from the American Statistical Association. In order to make a more scientific statistical decision in this case, a range of factors should be carefully considered to make a rational choice or adjustment to the value of α.
3. What should we do?
When the p-value is close to 0.05, there are three points for a decision-maker to consider. These points should jointly or individually be weighed against the p-value, and the final value of α should be determined or adjusted accordingly.
Effect size The first is whether the estimated value of θ indicates a strong effect. Suppose again p-value = 0.052 and the effect is found to be strong, then the researcher should reject H0, by choosing the value of α of 0.10 or higher. Conversely, if the effect is found to be negligible, then it is appropriate not to reject H0, by choosing the value of α of 0.01 or lower. When the effect is negligible, then it means that H0 is highly likely and it is reasonable to avoid Type I error by setting a low value for α. Conversely, if the effect is strong and H1 is highly likely, it is reasonable to avoid Type II error by setting a high value for α. This will reduce the value of β (probability of Type II error), as a result of the trade-off between α and β.
Relative loss of Type I and II errors Type I and II errors come with losses or consequences. Where the losses are known or estimable, the researchers should incorporate the relative loss into their decision-making. Suppose again p-value = 0.052. Let us assume that Type I error (rejecting the true H0) is going to incur a loss of $1M, while Type II error (accepting the false H0) will make little loss. Then, the researcher should avoid Type I error by controlling it with a low probability such as 0.01 or lower, i.e., by setting α ≤ 0.01. So, in this case, it is rational not to reject H0 at α = 0.01. Conversely, suppose the Type II error is much more costly than Type I error. Then, the researcher should increase the value of α to reduce the value of β and to avoid the Type II error. This is again on the basis of the above-mentioned trade-off between α and β. In this case, it is rational to set α ≥ 0.10 and reject H0.
Prior knowledge
Prior knowledge about the effect (θ) may exist in the form of
theories,
stylized facts (accumulated statistical evidence),
expert opinions and their consensus, or
common sense or intuition.
Suppose there is a theory or expert opinion, which strongly suggests the existence of a strong effect. In this case, when the p-value = 0.052, it is not reasonable to accept H0 at α = 0.05. When the prior knowledge strongly supports H1, then a high value of α should be chosen so that a low value is given to β. This is because, given that H1 is highly likely, the researcher wants to control Type II error with a low probability. Hence, in this case, it is reasonable to set α ≥ 0.10, and reject H0.
Conversely, if the prior knowledge strongly supports H0 of no effect, then the researcher should control Type I error with a lower probability by setting a lower value of α. This is because, given that H0 is highly likely, the researcher should avoid Type I error. Hence, in this case, it is reasonable to set α ≤ 0.01, and accept H0.
4. Examples
In this section, two example are presented as applications.
The first is the well-known example of spurious correlation between the number of drowning deaths in the U.S. against the number of movies Nicolas Cage appeared from 1999 to 2009. See this post for more details. Let Y be the number of deaths and X be the the number of movies Nicolas Cage appeared. For the regression Y = β0 + β1 X + u, the estimated slope coefficient is 5.82 with the t-statistic of 2.68 and (two-tailed) p-value of 0.025. Should we reject H0: β1 = 0 at the 5% level of significance or accept it at the 1% level of significance?
Suppose the researcher believes that there is little substantive importance to this relationship with a judgement that H0: β1 = 0 is highly likely to be true. She has further consulted a number of experts, who also support her judgement. Based on this, she wants to avoid Type I error by selecting a low probability for it, such as 0.01. That is, at α = 0.01, H0: β1 = 0 can not be rejected since p-value = 0.025 is greater than 0.01.
As the second example, we consider Example 17.10 of Selvanathan (2017). A production manager wishes to examine the relationship between aptitude test scores given prior to hiring and performance ratings of employees, three months after commencing work. From a random sample of 20 workers, the correlation (ρ) is calculated with the sample value r = 0.379. The Z statistic for H0:ρ = 0; H1: ρ ≠ 0, which follows the standard normal distribution, is given by
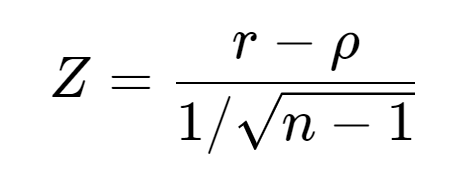
which gives the value of 1.652 under H0 with p-value = 0.098. Again, should the manager choose 0.05 or 0.10 level of significance?
Suppose the manager finds that r = 0.379 is not a negligible correlation, and it is widely regarded as a value of correlation representing a moderate association: see, for example, this post. Based on this, the manager decides that H1 is highly likely and sets α at a higher value such as 0.10 to control Type II error with a lower probability. As a result, H0 is rejected at the 10% level of significance in favour of H1 since p-value = 0.098 < 0.10.
In this post, I have explained how to adjust the level of significance, paying attention to the case where p-value is close to 0.05. There is little guidance about this documented elsewhere, and this post is aimed to provide data scientists several key points to consider, when they are in such a situation. A method of choosing the level of significance in a more formal and general setting can be found in this post.
The discussions above implicitly assume that α = 0.05 as an initial level of significance. But the same logic and argument should be applicable when the initial value of α is 0.10 or 0.01.
The main takeaway is that, when the researchers choose or adjust the level of significance, they should justify their choice considering the key factors including the
effect size,
relative loss from Type I and II errors, and/or
prior knowledge.
An arbitrary adjustment of the level of significance is unscientific and should be avoided.
Comments